对于线性回归, 成本函数为–
![J(\ Theta)= \ frac {1} {m} \ sum_ {i = 1} ^ {m} \ frac {1} {2} [h _ {\ Theta}(x ^ {(i)})-y ^ {(i)}] ^ {2}](https://www.lsbin.com/wp-content/uploads/2021/05/quicklatex.com-3716654884181f571d24bf33f1cb49bb_l3.png)
但是对于Logistic回归,

这将导致非凸成本函数。但这会导致成本函数具有局部最优值, 这对于梯度下降计算全局最优值来说是一个很大的问题。
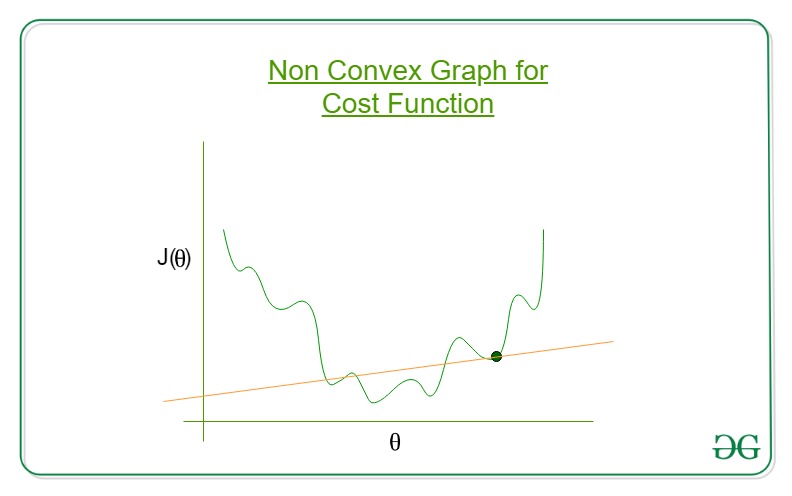
因此, 对于Logistic回归, 成本函数为

如果y = 1
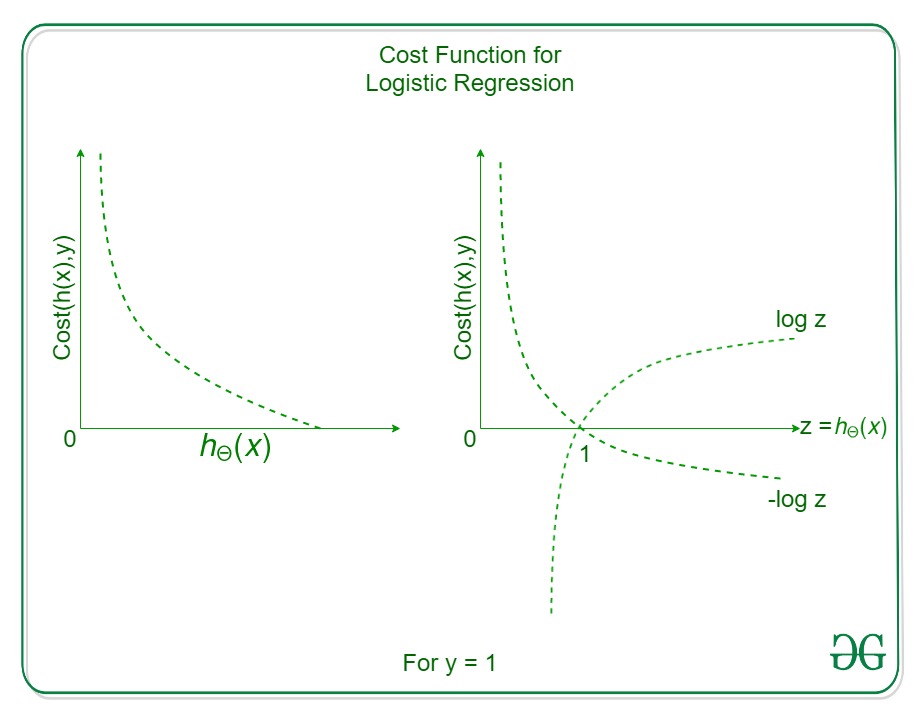
Cost = 0 if y = 1, hθ(x) = 1
但是
hθ(x) -> 0
Cost -> Infinity
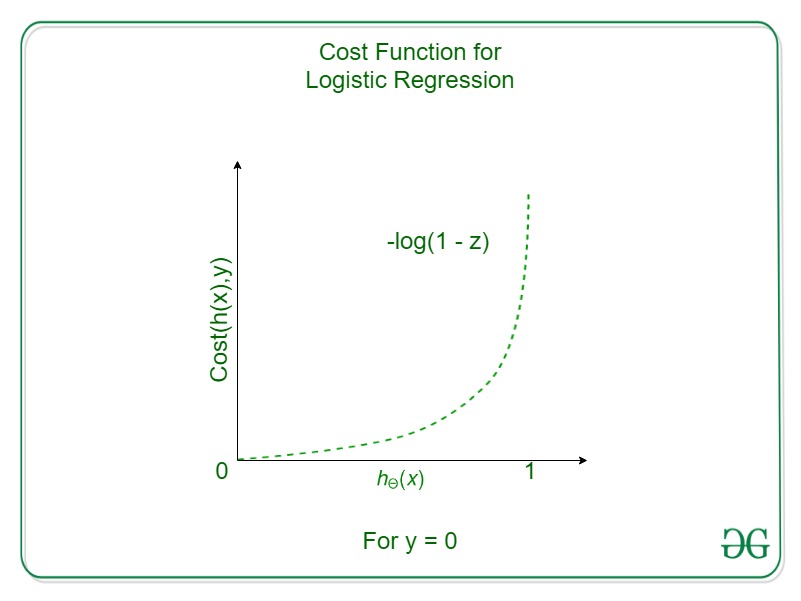
所以,



拟合参数θ, 必须将J(θ)最小化, 并且为此需要梯度下降。
梯度下降 -看起来与线性回归相似, 但区别在于假设hθ(X)

首先, 你的面试准备可通过以下方式增强你的数据结构概念:Python DS课程。