波士顿房屋数据:该数据集取自StatLib库, 并由卡内基梅隆大学维护。该数据集涉及房屋城市波士顿的房价。提供的数据集具有506个实例和13个特征。
数据集描述取自
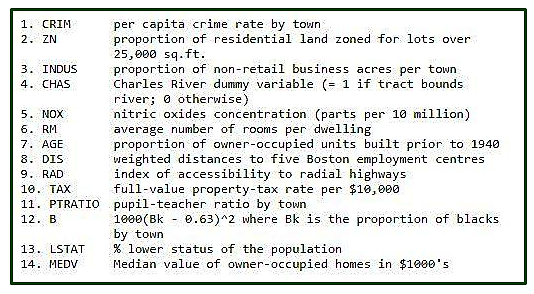
让我们建立线性回归模型, 预测房价
输入库和数据集。
# Importing Libraries
import numpy as np
import pandas as pd
import matplotlib.pyplot as plt
# Importing Data
from sklearn.datasets import load_boston
boston = load_boston()
输入波士顿数据的形状并获取feature_names
boston.data.shape

boston.feature_names

将数据从nd-array转换为dataframe并将特征名称添加到数据
data = pd.DataFrame(boston.data)
data.columns = boston.feature_names
data.head( 10 )
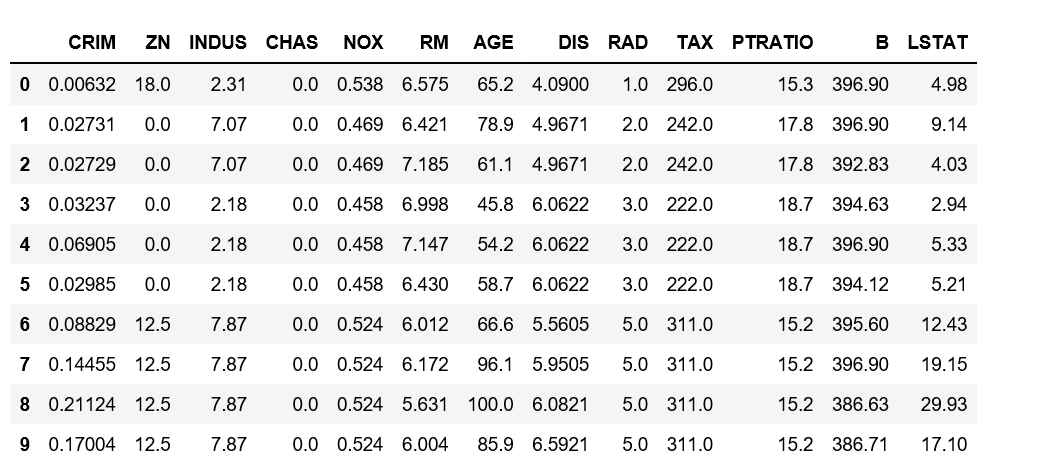
在数据集中添加"价格"列
# Adding 'Price' (target) column to the data
boston.target.shape

data[ 'Price' ] = boston.target
data.head()
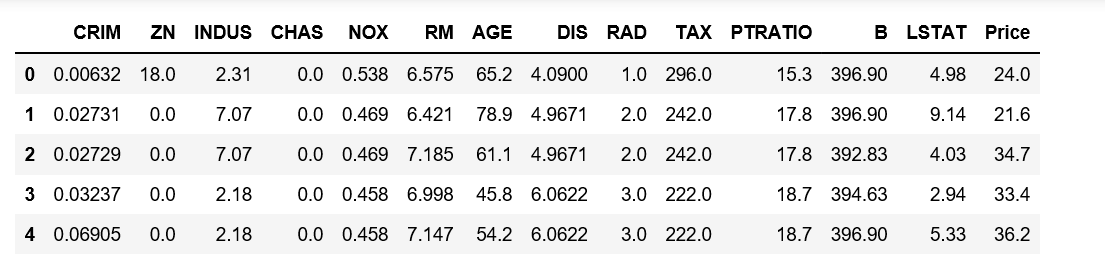
波士顿数据集的描述
data.describe()
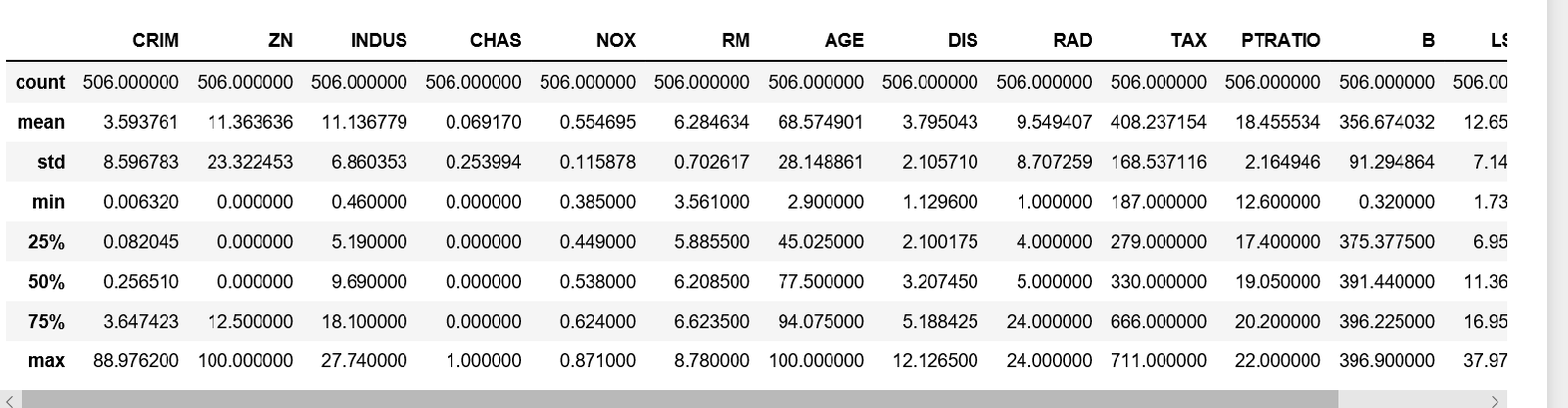
波士顿数据集的信息
data.info()
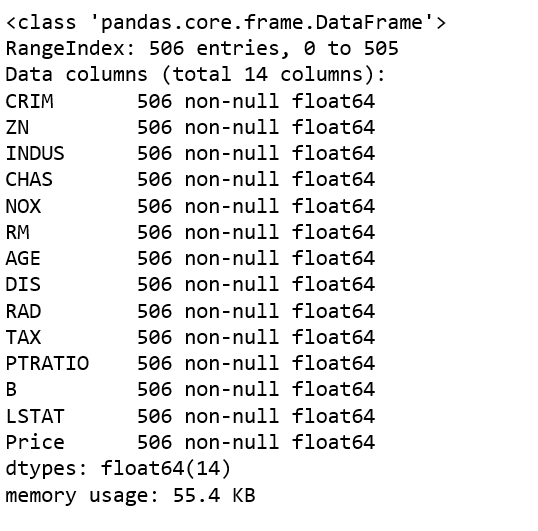
获取输入和输出数据, 并将数据进一步拆分为训练和测试数据集。
# Input Data
x = boston.data
# Output Data
y = boston.target
# splitting data to training and testing dataset.
from sklearn.cross_validation import train_test_split
xtrain, xtest, ytrain, ytest = train_test_split(x, y, test_size = 0.2 , random_state = 0 )
print ( "xtrain shape : " , xtrain.shape)
print ( "xtest shape : " , xtest.shape)
print ( "ytrain shape : " , ytrain.shape)
print ( "ytest shape : " , ytest.shape)
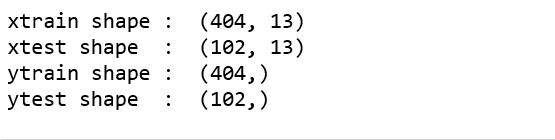
将线性回归模型应用于数据集并预测价格。
# Fitting Multi Linear regression model to training model
from sklearn.linear_model import LinearRegression
regressor = LinearRegression()
regressor.fit(xtrain, ytrain)
# predicting the test set results
y_pred = regressor.predict(xtest)
绘制散点图以显示预测结果-" ytrue"值与" y_pred"值
# Plotting Scatter graph to show the prediction
# results - 'ytrue' value vs 'y_pred' value
plt.scatter(ytest, y_pred, c = 'green' )
plt.xlabel( "Price: in $1000's" )
plt.ylabel( "Predicted value" )
plt.title( "True value vs predicted value : Linear Regression" )
plt.show()
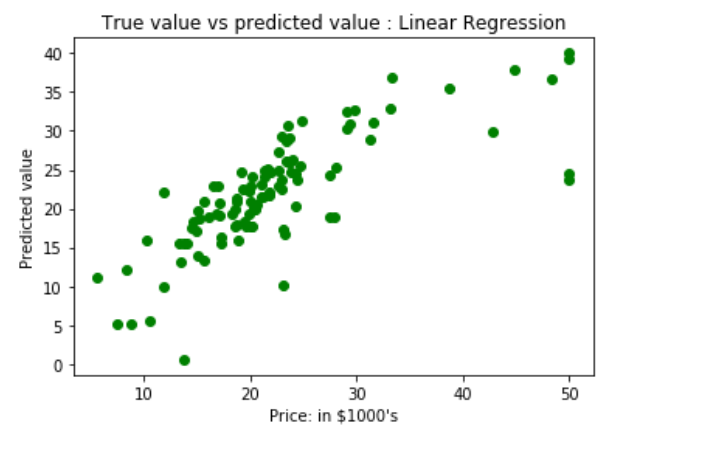
线性回归的结果, 即均方误差。
# Results of Linear Regression.
from sklearn.metrics import mean_squared_error
mse = mean_squared_error(ytest, y_pred)
print ( "Mean Square Error : " , mse)

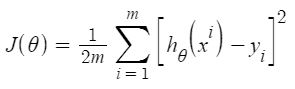
根据结果, 我们的模型只有66.55%的准确度。因此, 准备好的模型对于预测房屋价格不是很好。可以使用许多其他可能的机器学习算法和技术来改善预测结果。
首先, 你的面试准备可通过以下方式增强你的数据结构概念:Python DS课程。